What Clinicians and Administrators Need to Know When Implementing AI-The HSB Blog 9/13/21
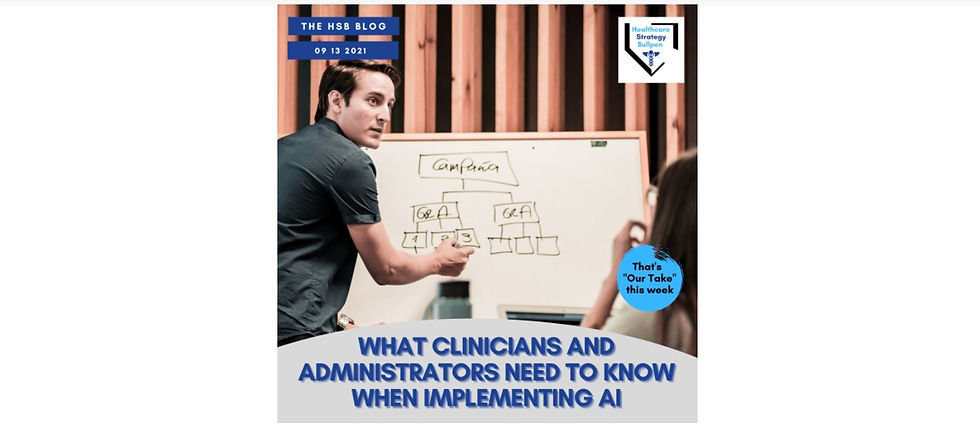
Our Take:
There are several basic issues and challenges in deploying AI that all clinicians and administrators should be aware of and inquire about to ensure that they are being properly being considered when AI is being implemented in their organization. Applications of artificial intelligence in healthcare hold great promise to increase both the scale of medical discoveries and the efficiency of healthcare infrastructure. As such healthcare-related research and investment has exploded over the last several years. For example, according to the State of AI Report 2020, academic publications in biology around AI technologies such as deep learning, natural language processing (NLP), and computer vision have grown over 50% a year since 2017. In addition, 99% of healthcare institutions surveyed by CB Insights are either currently deploying (38%) or planning to deploy AI (61%) in the near future. However, as witnessed by recent errors discovered surrounding the application of an AI-based Sepsis model, while AI can improve quality of care, improve access and reduce costs, models must be implemented correctly or they will be of questionable value and even dangerous.
Key Takeaways:
According to Accenture’s “Artificial Intelligence: Healthcare’s New Nervous System” report, AI for health is expected to grow at a 40% CAGR through 2021.
Researchers working to uncover insights into prescribing patterns for certain antipsychotic medications found that approximately 27% of prescriptions were missing dosages.
Even after doing work to standardize and label patient data, in at least one broad study almost 10% of items in the data repository didn’t have proper identifiers.
Academic publications in biology around AI technologies such as deep learning, natural language processing (NLP), and computer vision have grown over 50% a year since 2017.
The Problem:
While it is commonly accepted that computers can outperform humans in terms of computational speed, in its current state many would argue that artificial intelligence is really “augmented intelligence” defined by the IEEE as “a subsection of AI machine learning developed to enhance human intelligence rather than operate independently of or outright replace it.” Current AI models are still highly dependent upon the quantity and quality of data available for them to be trained on, the inherent assumptions underlying the models as well as the human biases (intentional and unintentional) of those developing the models along with a number of other factors. As noted in a recent review of the book “I, Warbot” about computational warfare by Kings College, AI lecturer Kenneth Payne, “these gizmos exhibit ‘exploratory creativity'-essentially a brute force calculation of probabilities. That is fundamentally different from ‘transformational creativity”, which entails the ability to consider a problem in a wholly new way and requires playfulness, imagination and a sense of meaning.” As such, those creating AI models for healthcare need to ensure they set the guardrails for its use and audit its models both pre and post-development to ensure they conform to existing laws and best practices.
The Backdrop:
When implementing an AI project there are a number of steps and considerations that should be taken into account to ensure its success. While it is important to identify the best use and type with any kind of project, given the cost of the technical talent involved, the level of computational infrastructure typically needed (if done internally) and the potential to influence leadership attitudes towards the use and viability of AI as an organizational tool, it is even more important here. As noted above one of the most important keys to implementing an AI project is the quantity and quality of data resources available to the firm. Data should be looked at with respect to both quality (to ensure that it is free of missing, incoherent, unreliable, or incorrect values) and quantity. In terms of data quality, as noted in “Artificial Intelligence: A Non-Technical Introduction”, data can be: 1) noisy (have data sets with conflicting data), 2) dirty (have data sets with inconsistent and erroneous data), 3) sparse (have data with missing or no values at all, or, 4) inadequate (have data sets that have contained inadequate or biased data).
As noted in an article in “Extracting and Utilizing Electronic Health Data from Epic for Research”, “to provide the cleanest and most robust datasets for statistical analysis, numerous statistical techniques including similarity calculations and fuzzy matching are used to clean, parse, map, and validate the raw EHR data.” which is generally the largest source of healthcare data for AI research. When looking to implement AI it is important to consider and understand the levels of data loss and the ability to correct for it. For example, researchers looking to apply AI to uncover insights into prescribing patterns into second-generation antipsychotic medications (SGAs) found that approximately 27% of the prescriptions in their data set were missing dosages and even after undertaking a 3-step correction procedure, 1% were missing dosages. While this may be deemed an acceptable number it is important to be aware of the data loss and know this information in order to properly evaluate if it is within tolerable limits.
In terms of inadequate data, ensuring that data is free of bias is extremely important. While we have all recently been made keenly aware of the impact of racial and ethnic bias on models (ex: facial recognition models trained only on Caucasians) there are a number of other biases which models should be evaluated for. According to “7 Types of Data Bias in Machine Learning” these include: 1) sample bias (not representing the desired population accurately), 2) exclusion bias (the intentional or unintentional exclusion or certain variables from data prior to processing), 3) measurement bias (ex: due to poorly chosen measurements that create systematic distortions of data, like poorly phrased surveys); 4) recall bias (when similar data is inconsistently labeled), 5) observer bias ( when the labelers of data let their personal views influence data classification/annotation), 6) racial bias (when data samples skew in favor of or against certain ethnic or demographic groups), 7) association bias (when a machine learning model reinforces a bias present in a model).
In addition to data quality, data quantity is as imperative. For example, in order to properly train machine learning models, you need to have a sufficiently large number of observations to create an accurate predictor of the parameters you’re trying to forecast. While the precise number of observations needed will vary based on the complexity of the data you’re using, the complexity of the model you want to build, and the impact of the amount of “statistical noise” generated by the data itself, an article in the Journal of Machine Learning Research suggested that at least 100,000 observations are needed to train a regression or classification model. Moreover, it is important that numerous data points are not captured or sufficiently documented in healthcare. For example, as noted in the above-referenced article on extracting and utilizing Epic EHR data for study based on research at the Cleveland Clinic in 2018, even after doing significant work to standardize and label patient data, “approximately 9% [1,000 out of 32,000 data points per patient] of columns in the data repository” were not using the assigned identifiers. While it is likely that methods have improved since this research was performed, given the size and resources that an institution like the Cleveland Clinic had to bear on the problem, it indicates the larger size of the problem.
Once the model has been developed there should be a process in place to ensure that the model is transparent and explainable by creating a mechanism that allows non-technologists to understand and assess the factors the model used and what parameters it relied most heavily upon in coming to its conclusions. For example, as noted by the State of AI Report 2020, “AI research is less open than you think, only 15% of papers publish their [algorithmic] code” used to weight and create models. In addition, there should be a system of controls, policies, and audits in place that provide feedback as to the potential errors in the application of the model as well as disparate impact or bias in its conclusions.
Implications:
As noted in “Artificial Intelligence Basics: A Non-Technical Introduction” it’s important to have realistic expectations for what can be accomplished by an AI project and how to plan for it. In the book, the author Andrew Taulli references Andrew Ng, the former Head of Google Brain, who suggests the following parameters; an AI project should take between 6-12 months to complete, have an industry-specific focus, should notably help the company, doesn’t have to be transformative, and, have high-quality data points. In our opinion, it is particularly important to form collaborative, cross-platform teams of data scientists, physicians, and other front-line clinicians (particularly those closest to patients like nurses) to get as broad input on the problem as possible. While AI holds great promise, proponents will have to prove themselves by running targeted pilots and should be careful not to overreach at the risk of poisoning the well of opportunity. As so astutely pointed out in “5 Steps for Planning A Healthcare Artificial Intelligence Project: “artificial intelligence isn’t something that can be passively infused into an organization like a teabag into a cup of hot water. AI must be deployed carefully, piece by piece, in a measured and measurable way.”
Data scientists need to ensure that the models they create produce relevant output that provide context and the ability for clinicians to have a meaningful impact upon the results and not just generate additional alerts that will go unheeded. For example, as Rob Bart, Chief Medical Information Officer at UPMC noted in a recent presentation at HIMSS, data should provide “personalized health information, personalized data” and should have “situational awareness in order to turn data into better consumable information for clinical decision making” in healthcare. Along those lines, it is important to take a realistic assessment of “where your organization lies on the maturity curve”, how good is your data, how deep is your bench of data scientists and clinicians available to work on an AI project in order to inventory, clean and prepare your data. AI talent is highly compensated and in heavy demand. Do you have the resources necessary to build and sustain a team internally or will you need to hire external consultants? How will you select and manage those consultants, etc.? All of these are questions that need to be carefully considered and answered before undertaking the project. In addition, healthcare providers need to consider the special relationship between clinician and patient and the need to preserve trust, transparency, and privacy. While AI holds a tremendous allure for healthcare and the potential for it to overcome, and in fact make up for its underinvestment in information technology relative to other industries, all of this needs to be done with a well-thought-out, coherent and justified strategy as its foundation.
Related Readings:
Comments